Algorithmic trading, often referred to as algo trading or automated trading, is a cutting-edge approach to executing trades in financial markets. It involves the use of computer algorithms to generate and execute trading signals based on predefined parameters, eliminating human emotion and bias from the decision-making process. This powerful tool has gained significant traction in recent years, transforming the landscape of financial markets.
Algorithmic trading offers a multitude of advantages, including:
Enhanced Efficiency: Algorithms can execute trades at optimal prices and manage portfolios with unparalleled efficiency, maximizing potential returns while minimizing costs.
Reduced Emotional Bias: The absence of emotional involvement ensures that trading decisions are made based on data and algorithms rather than emotions, reducing the impact of impulsive or irrational choices.
Risk Management: Algorithmic trading systems can incorporate risk management parameters to limit potential losses, ensuring that trading strategies align with predefined risk tolerances.
By harnessing the power of algorithms and data, algorithmic trading has the potential to revolutionize the way we approach investing, offering a more objective and data-driven approach to market participation.
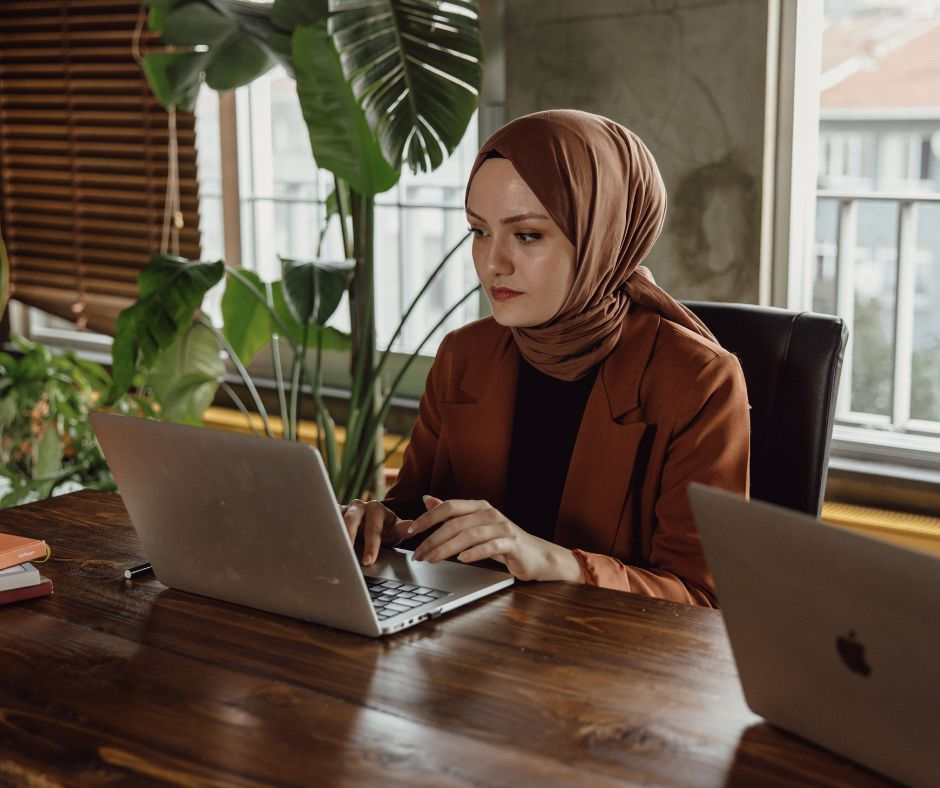
Definition of Algorithmic Trading
Algorithmic trading, often referred to as algo trading or automated trading, is a cutting-edge method that harnesses advanced mathematical models and computer algorithms to execute a high volume of financial transactions at incredible speeds. These algorithms are designed to make real-time decisions regarding the buying and selling of financial instruments, such as stocks, bonds, commodities, or currencies. Algorithmic trading encompasses a wide array of strategies, from simple to highly complex, each aiming to optimize trading performance and profitability.
Benefits of Algorithmic Trading
Algorithmic trading comes with a myriad of benefits, making it a highly attractive option for traders and investors:
Increased Efficiency:
Algorithms can execute trades at optimal prices and manage portfolios with unparalleled efficiency, maximizing potential returns while minimizing costs.
Reduced Emotional Bias:
The absence of emotional involvement ensures that trading decisions are made based on data and algorithms rather than emotions, reducing the impact of impulsive or irrational choices.
Risk Management:
Algorithmic trading systems can incorporate risk management parameters to limit potential losses, ensuring that trading strategies align with predefined risk tolerances.
By understanding the significance of algorithmic trading in today's financial landscape and how it diverges from traditional trading methods, you'll be better equipped to appreciate the advantages it offers in the world of personal finance and investing.
Key Components of Algorithmic Trading
In the world of algorithmic trading, success hinges on a well-structured system that encompasses several key components. This section delves into these essential elements, highlighting the significance of data, strategies, and execution in algorithmic trading. Additionally, we'll explore the vital roles that historical data analysis and risk management play in ensuring the effectiveness of algorithmic trading strategies.
Data: The Foundation of Algorithmic Trading
At the core of any algorithmic trading system lies data. The success of an algorithmic strategy depends on the quality and availability of data, which can include historical price and volume data, news feeds, economic indicators, and more. These data sources serve as the foundation for decision-making in algorithmic trading.
Historical Data: Historical data analysis is indispensable for understanding market patterns, identifying trends, and developing strategies. This historical data can encompass price and volume information, as well as other relevant market metrics.
Real-time Data: Real-time data feeds keep algorithms up to date with the latest market conditions, ensuring that decisions are made based on the most current information available.
Strategies: Crafting the Blueprint for Trading
Algorithmic trading strategies are the blueprints that guide the system's decision-making process. Strategies can range from simple, rules-based approaches to highly complex machine learning models. The choice of strategy depends on the trader's objectives and the assets being traded.
Trend Following: Strategies that identify and capitalize on market trends, buying when prices are rising and selling when they are falling.
Mean Reversion: Strategies that seek to profit from the tendency of prices to revert to their historical average.
Arbitrage: Strategies that exploit price differences in related assets or markets, aiming to capture risk-free profits.
Execution: Turning Strategies into Action
Once a strategy is developed, the execution phase comes into play. This involves implementing the trading decisions generated by the algorithm in a way that minimizes market impact and maximizes efficiency.
Order Routing: Deciding how and where to place orders in the market to achieve the desired execution.
Algorithm Configuration: Optimizing the algorithm's parameters to ensure it aligns with the trader's objectives and market conditions.
Historical Data Analysis: The Informed Foundation
Historical data analysis is the cornerstone of algorithmic trading. By meticulously examining past market behavior, traders gain insights into price patterns, asset correlations, and the effectiveness of various strategies. This historical analysis informs the design and optimization of trading strategies, improving the likelihood of success.
Backtesting: The process of applying a trading strategy to historical data to evaluate its performance. Backtesting helps traders refine strategies and assess their viability.
Machine Learning Models: Leveraging historical data to train machine learning models to make predictions and inform trading decisions.
Risk Management: Preserving Capital
In algorithmic trading, risk management is paramount. It involves implementing controls and safeguards to protect capital and minimize losses.
Position Sizing: Determining the size of each trade to limit exposure and adhere to predefined risk tolerances.
Stop Loss Orders: Automatically exiting a trade when it reaches a predetermined loss threshold to mitigate potential losses.
Diversification: Spreading investments across different assets and markets to reduce the impact of adverse movements in any single asset.
An effective algorithmic trading system combines data, strategies, and execution seamlessly while drawing insights from historical data and safeguarding investments through robust risk management practices. These components work in concert to enhance the probability of success in algorithmic trading.
Types of Algorithmic Trading Strategies
Algorithmic trading offers a diverse array of strategies, each tailored to different market conditions and objectives. In this section, we'll explore various types of algorithmic trading strategies, providing examples and explanations for each one. Understanding the differences between these strategies is crucial because they are best suited for specific market conditions.
Trend Following Strategies
Trend following strategies aim to identify and capitalize on prevailing market trends. Traders following this approach buy assets when prices are rising and sell when they're falling, with the expectation that trends will continue. Example: Moving Average Crossover
In this strategy, two moving averages are used, typically a short-term and a long-term one.
When the short-term moving average crosses above the long-term moving average, it generates a buy signal.
Conversely, when the short-term moving average crosses below the long-term moving average, it generates a sell signal.
Trend following strategies are best suited for trending markets, where asset prices exhibit consistent upward or downward movements.
Mean Reversion Strategies
Mean reversion strategies assume that prices tend to revert to their historical average over time. These strategies are based on the belief that when prices deviate significantly from their average, they are likely to correct. Example: Bollinger Bands
Bollinger Bands consist of a moving average (the mean) and upper and lower bands that are a certain number of standard deviations away from the mean.
When an asset's price reaches the upper band, it's considered overbought, and a sell signal is generated.
Conversely, when the price touches the lower band, it's considered oversold, and a buy signal is generated.
Mean reversion strategies are most effective in range-bound markets, where prices oscillate within a certain range.
Arbitrage Strategies
Arbitrage strategies involve exploiting price differences in related assets or markets to generate risk-free profits. These price differences can occur due to market inefficiencies or temporal lags. Example: Statistical Arbitrage
In this strategy, a trader simultaneously buys and sells two correlated assets when they deviate from their historical relationship.
For instance, if Stock A and Stock B typically move in tandem, but Stock A suddenly experiences a price drop while Stock B doesn't, the trader buys Stock A and sells Stock B with the expectation that the price relationship will eventually revert to its historical mean.
Arbitrage strategies thrive in markets with inefficiencies, where prices of related assets are temporarily mispriced.
High-Frequency Trading (HFT) Strategies
High-frequency trading strategies involve executing a large number of trades within milliseconds. These strategies exploit tiny price differentials that can occur in fast-moving markets. Example: Market Making
Market makers provide liquidity to the market by continuously quoting buy and sell prices for an asset.
They profit from the bid-ask spread by buying at the bid price and selling at the ask price.
HFT strategies are most effective in highly liquid markets where rapid price changes offer numerous trading opportunities.
Sentiment Analysis Strategies
Sentiment analysis strategies use natural language processing and machine learning to gauge market sentiment from news articles, social media, or other sources. They aim to predict market movements based on collective investor sentiment. Example: Twitter Sentiment Analysis
Analyzing Twitter data to gauge public sentiment about a particular stock or asset.
Positive sentiment might lead to a buy signal, while negative sentiment could trigger a sell signal.
Sentiment analysis strategies are useful when market sentiment has a significant impact on asset prices, making them most effective in news-driven markets. Each type of algorithmic trading strategy has its strengths and weaknesses, and choosing the right one depends on the prevailing market conditions and the specific objectives of the trader or investor. By understanding these strategies, you can make more informed decisions about which one aligns with your investment goals and the market conditions you're facing.
Building Your Own Algorithmic Trading System
Creating a personal algorithmic trading system can be a rewarding endeavor, but it requires careful planning and execution. In this section, we will explore the steps involved in developing your algorithmic trading system, emphasizing the significance of backtesting and optimization. We'll also provide resources and tools to assist individuals looking to embark on this journey.
Steps to Develop Your Algorithmic Trading System
Building your algorithmic trading system involves several key steps:
Define Your Strategy: Start by defining your trading strategy, whether it's a trend-following, mean-reversion, or another approach. Consider your risk tolerance, time horizon, and target assets.
Data Acquisition: Acquire the necessary historical and real-time data for your chosen assets. This data will be used for analysis, testing, and live trading.
Coding and Algorithm Development: Write the code for your trading algorithm. You may use programming languages like Python, R, or specialized trading platforms that offer algorithm development capabilities.
Backtesting: Backtest your algorithm by running it against historical data to evaluate its performance. This step is crucial for refining your strategy and identifying potential issues.
Optimization: Use the insights gained from backtesting to optimize your algorithm. Adjust parameters and fine-tune the strategy for better results.
Risk Management: Implement risk management techniques, including position sizing, stop-loss orders, and portfolio diversification, to protect your capital.
Broker and Platform Selection: Choose a reliable brokerage platform and connect it to your algorithm. Ensure that it supports your preferred assets and order types.
Paper Trading: Before risking real capital, engage in paper trading (simulated trading) to test your algorithm in a risk-free environment.
Live Trading: Once you are confident in your algorithm's performance during paper trading, consider transitioning to live trading with real capital.
The Importance of Backtesting and Optimization
Backtesting is the process of applying your trading strategy to historical data to assess its performance. This step is crucial for several reasons:
Refinement: Backtesting helps you identify flaws or weaknesses in your strategy, allowing you to refine it before risking real capital.
Historical Perspective: It provides insight into how your strategy would have performed in the past, giving you a sense of its potential in different market conditions.
Data-Driven Decisions: Backtesting allows you to make data-driven decisions and optimize your strategy for better risk-adjusted returns.
Optimization is the natural next step after backtesting. It involves fine-tuning your algorithm based on the insights gained from the backtest results. By adjusting parameters, optimizing execution rules, and applying other refinements, you can enhance the algorithm's potential for success.
Resources and Tools for Algorithm Development
For individuals looking to create their algorithmic trading systems, there are a variety of resources and tools available:
Programming Languages: Python is a popular choice for algorithmic trading due to its extensive libraries and ease of use. R is another option, particularly for data analysis.
Algorithmic Trading Platforms: Platforms like MetaTrader, NinjaTrader, and QuantConnect offer tools for algorithm development, backtesting, and live trading.
Data Sources: Access historical and real-time data from sources like Quandl, Alpha Vantage, Yahoo Finance, and dedicated data providers.
Educational Resources: Online courses, books, and forums on algorithmic trading, quantitative finance, and programming can help you gain the necessary knowledge and skills.
Community Support: Engage with online communities, such as Quantitative Finance Stack Exchange or trading forums, to seek advice and share experiences with fellow algorithmic traders.
Building your own algorithmic trading system requires dedication, a strong foundation in financial markets, programming skills, and a willingness to continually learn and adapt. By following the steps outlined, using backtesting and optimization effectively, and leveraging available resources, you can develop a robust trading system tailored to your specific goals and preferences.
Risks and Challenges of Algorithmic Trading
While algorithmic trading offers numerous advantages, it is not without its risks and challenges. In this section, we'll delve into potential pitfalls associated with algorithmic trading, including technical failures and market volatility. We'll also explore strategies for mitigating these risks and managing losses. Additionally, we'll touch on regulatory considerations and ethical concerns that traders should be mindful of.
Potential Risks in Algorithmic Trading
Technical Failures:
Algorithmic trading systems are highly reliant on technology, and technical failures can be catastrophic. These failures can result from software glitches, hardware malfunctions, connectivity issues, or even cyberattacks.
Market Volatility:
Rapid and unexpected market movements, often associated with events like economic reports, geopolitical incidents, or extreme weather events, can lead to substantial losses if algorithms fail to adapt or properly manage risk.
Execution Risk:
Poor execution of orders, whether due to a lack of liquidity or slippage, can lead to unfavorable prices and significant financial losses.
Model Risk:
Overreliance on historical data and assumptions within algorithmic models can lead to errors and losses, especially if market conditions change.
Mitigating Risks and Managing Losses
Diversification:
Spreading investments across different assets, markets, and strategies can help mitigate losses from adverse price movements in a single asset or market.
Risk Management:
Implement strict risk management measures, such as setting stop-loss orders, position sizing, and portfolio rebalancing. These measures help protect your capital from significant losses.
Testing and Monitoring:
Regularly test algorithms in different market conditions and monitor their performance. Be prepared to intervene if anomalies or irregularities are detected.
Redundancy and Contingency Plans:
Implement redundancy in your technology infrastructure and have contingency plans in place to address technical failures. This includes backup systems, data feeds, and alternative execution options.
Model Validation:
Continuously validate and update your algorithms to ensure they remain effective in changing market conditions.
Regulatory Considerations and Ethical Concerns
Regulatory Considerations:
Algorithmic trading is subject to regulations designed to ensure market integrity and investor protection. Traders and institutions engaged in algorithmic trading should adhere to local financial regulations and reporting requirements.
Market Manipulation:
Algorithmic trading can, in some cases, be used to manipulate markets or exacerbate price movements. This presents ethical concerns and legal risks. Traders should operate ethically and in accordance with market regulations.
Market Surveillance:
Regulators employ surveillance tools to monitor trading activities, particularly in high-frequency trading. Traders should be aware of these monitoring systems and act within regulatory guidelines.
Data Privacy:
The use of data, including market data and customer data, must comply with data privacy regulations and ethical standards. Protecting sensitive information and ensuring data security is crucial. Algorithmic trading is a powerful tool, but it is not without risks. It requires a thoughtful and diligent approach to technology, risk management, and compliance. By understanding the potential risks and taking proactive steps to mitigate them, traders can harness the benefits of algorithmic trading while safeguarding their financial well-being and maintaining ethical standards in the market.
Conclusion:
Algorithmic trading has emerged as a transformative force in the financial world, offering a sophisticated and efficient approach to trading. By leveraging the power of algorithms, data, and risk management, algorithmic trading has the potential to enhance portfolio performance, mitigate risks, and unlock new opportunities for investors.
As algorithmic trading continues to evolve, it is poised to play an even more prominent role in the financial landscape. Those who embrace its principles and adapt to its advancements will be well-positioned to navigate the complexities of the market and achieve their investment goals.
Comments