Machine learning has become a transformative force in the finance sector, revolutionizing the way financial institutions operate, make decisions, manage risks, and formulate strategies. This article delves into the profound significance of machine learning in finance, exploring its far-reaching impacts on key aspects such as decision-making, risk management, and overall financial strategies.
The finance industry has long been data-driven, with the need to process vast amounts of information for decision-making and risk assessment. Machine learning, a subset of artificial intelligence, has emerged as a game-changer in this domain, owing to its ability to analyze vast datasets and extract valuable insights, thereby empowering financial institutions to make more informed and strategic choices.
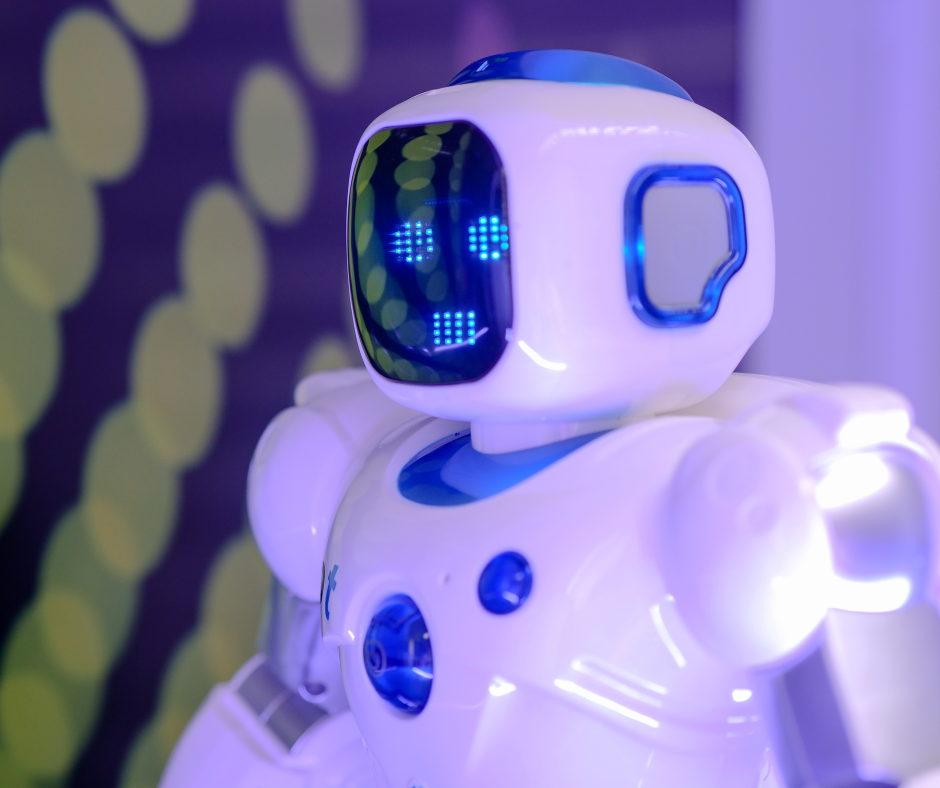
Understanding Machine Learning in Finance
Defining Machine Learning and Its Relevance in the Financial Industry
Machine learning is a subset of artificial intelligence that focuses on the development of algorithms and statistical models which enable computers to learn and make predictions or decisions without being explicitly programmed. In the financial industry, machine learning plays a crucial role in automating processes, extracting insights from vast datasets, and improving decision-making through data-driven models. Its relevance in finance stems from its ability to handle complex, large-scale data, identify patterns, and make predictions with a high degree of accuracy.
Examples of Financial Tasks Enhanced by Machine Learning
Machine learning has the potential to automate and enhance a wide range of financial tasks, including:
Algorithmic Trading: Machine learning algorithms can analyze historical market data, identify patterns, and execute trades in real-time, taking advantage of short-term market opportunities.
Credit Risk Assessment: Financial institutions use machine learning to assess credit risk by analyzing an applicant's credit history, income, and other factors. This enables more accurate and timely lending decisions.
Fraud Detection: ML models can detect unusual patterns and anomalies in transaction data, helping to identify and prevent fraudulent activities in real-time.
Customer Segmentation: By analyzing customer data, machine learning can segment clients based on behavior, preferences, and demographics, allowing financial institutions to offer personalized products and services.
Portfolio Management: ML algorithms can optimize investment portfolios by continuously monitoring market conditions and adjusting asset allocations to maximize returns while managing risk.
How Machine Learning Differs from Traditional Financial Analysis?
Machine learning differs from traditional financial analysis in several key ways:
Data-Driven Decision-Making: Traditional financial analysis often relies on historical financial statements, expert opinions, and fundamental analysis. Machine learning, on the other hand, leverages vast datasets, including real-time market data, news sentiment, and alternative data sources, to make data-driven predictions and decisions.
Automation: Machine learning automates many tasks that were previously performed by humans, such as stock trading, credit scoring, and fraud detection. This automation not only increases efficiency but also reduces human bias in decision-making.
Complex Pattern Recognition: Machine learning excels at identifying complex and non-linear patterns in data that might be challenging for traditional methods. It can process a vast amount of information quickly and adapt to changing market conditions.
Real-Time Analysis: Traditional financial analysis often involves periodic reviews and reports. Machine learning provides real-time analysis, allowing for immediate reactions to market changes and events.
Scalability: Machine learning can scale to handle large datasets and complex models, making it suitable for the ever-growing data requirements of the finance sector.
In summary, machine learning's ability to process vast amounts of data, automate tasks, and recognize complex patterns sets it apart from traditional financial analysis. Its role in the financial industry is pivotal, as it empowers institutions to make data-driven decisions, automate processes, and adapt to the dynamic nature of the financial markets.
The 12 Machine Learning Applications in Finance
Machine learning algorithms analyze historical market data, news sentiment, and other factors to make high-frequency trading decisions.
Real-World Example: Renaissance Technologies is known for using ML-driven strategies in their Medallion Fund to achieve exceptional returns.
Credit Risk Assessment:
Machine learning models evaluate a borrower's creditworthiness by considering factors such as credit history, income, and demographics.
Real-World Example: LendingClub uses machine learning to assess credit risk for personal loans, resulting in more accurate lending decisions.
Fraud Detection:
ML detects fraudulent activities in real-time by identifying anomalies and suspicious patterns in transaction data.
Real-World Example: PayPal uses machine learning to combat fraud, reducing false positives and saving millions in losses.
Customer Segmentation:
ML clusters customers based on behavior, preferences, and demographics to personalize product and service offerings.
Real-World Example: Amazon uses ML to segment its customers for tailored product recommendations.
Portfolio Management:
Machine learning optimizes investment portfolios by continuously monitoring market conditions and adjusting asset allocations.
Real-World Example: Wealthfront employs ML to provide automated, algorithm-driven portfolio management to its clients.
Predictive Analytics:
ML models forecast financial market trends and asset price movements based on historical data and external factors.
Real-World Example: Hedge funds like Two Sigma use predictive analytics models to make informed investment decisions.
Sentiment Analysis:
ML algorithms analyze news articles, social media, and other sources to gauge market sentiment and make trading decisions.
Real-World Example: Bloomberg incorporates sentiment analysis to provide traders with real-time market sentiment indicators.
Options Pricing:
Machine learning is used to refine options pricing models, enhancing accuracy in determining option values.
Real-World Example: Citadel Securities applies ML to options market-making strategies.
ML-driven robo-advisors create and manage diversified investment portfolios for clients, often with lower fees than traditional advisors.
Real-World Example: Betterment and Wealthsimple are robo-advisors that utilize machine learning to optimize portfolios.
Regulatory Compliance:
Machine learning assists in monitoring and ensuring compliance with financial regulations, reducing risks associated with non-compliance.
Real-World Example: Promontory Financial Group employs ML to enhance compliance and risk management services.
Trading Strategy Optimization:
ML models identify and adapt trading strategies to changing market conditions, aiming to maximize returns.
Real-World Example: WorldQuant, a quantitative investment firm, relies on machine learning to optimize trading strategies.
Anomaly Detection in Market Data:
ML algorithms detect unusual patterns and potential anomalies in market data, helping to uncover insider trading and irregularities.
Real-World Example: Nasdaq uses machine learning for surveillance and monitoring of market activities.
Benefits of These Applications:
Improved Accuracy:
Machine learning enhances decision-making by analyzing vast datasets and identifying subtle patterns that humans might miss, resulting in more accurate predictions and assessments.
Efficiency:
Automation of tasks such as trading, risk assessment, and fraud detection reduces operational costs and speeds up processes, enabling institutions to respond swiftly to market changes.
Personalization:
Customer segmentation and robo-advisors use machine learning to offer personalized financial products and services, improving customer satisfaction and loyalty.
ML models bolster risk assessment and regulatory compliance, helping financial institutions better manage and mitigate risks.
Cost Savings:
Detecting fraud and irregularities in real-time, with high accuracy, saves institutions substantial amounts of money that would otherwise be lost to fraudulent activities.
In conclusion, machine learning has revolutionized the finance sector by enhancing decision-making, risk assessment, and automation across various applications. Real-world examples demonstrate how these technologies are being successfully implemented to improve accuracy, efficiency, and profitability in the financial industry.
Challenges and Limitations
Challenges Faced in Implementing Machine Learning in Finance
Data Privacy Concerns:
Financial institutions handle sensitive customer data, making data privacy a paramount concern. Using machine learning in finance requires rigorous data protection and adherence to regulations like GDPR and HIPAA.
Model Interpretability:
Many machine learning models, such as deep neural networks, are often seen as "black boxes." Understanding the reasoning behind a model's decisions is crucial in finance, where accountability is essential. Interpretable AI techniques are still an ongoing area of research.
Bias and Fairness:
Machine learning models can inherit biases from training data, which can lead to unfair or discriminatory outcomes. In finance, biased models can result in unfair lending practices or skewed investment decisions.
Regulatory Hurdles:
Financial institutions must comply with a multitude of regulations. Implementing machine learning models that meet these regulatory requirements can be a significant challenge. Regulatory bodies are also working on defining frameworks for AI in finance.
Operational Risks:
Relying heavily on automation through machine learning can introduce operational risks, especially when models encounter unforeseen situations or anomalies that they are ill-equipped to handle.
Limitations and Potential Risks of Overreliance on Machine Learning Models
Overfitting:
Machine learning models can be overfit to historical data, leading to poor generalization to new, unseen data. Overreliance on such models can result in financial losses when markets deviate from historical patterns.
Data Quality:
Models are only as good as the data they are trained on. Poor data quality can lead to incorrect predictions and decisions. Garbage in, garbage out.
Market Shocks:
Machine learning models, like all predictive tools, are not immune to market shocks or extreme events that deviate significantly from historical data. Relying too heavily on models in such cases can lead to substantial losses.
Adversarial Attacks:
In high-stakes financial environments, malicious actors may attempt to manipulate machine learning models. If these models are not designed with robustness in mind, they can be vulnerable to attacks.
Examples of Machine Learning Failures in Finance
On May 6, 2010, the U.S. stock market experienced a sudden and severe crash, partly triggered by algorithmic trading programs. These programs, which heavily relied on machine learning models, exacerbated the market's volatility.
In 2012, Knight Capital Group lost over $450 million in less than an hour due to a software glitch in their algorithmic trading system. Machine learning models were used in their trading algorithms, and a flawed model update led to disastrous consequences.
Before the 2008 financial crisis, machine learning models were used to evaluate the risk of mortgage-backed securities and credit default swaps. These models largely failed to predict the impending financial meltdown.
These examples illustrate the risks associated with overreliance on machine learning in financial applications and emphasize the need for a careful and thoughtful approach to model development, risk management, and human oversight. While machine learning has the potential to offer significant benefits, it should be integrated into finance with a strong understanding of its limitations and a focus on risk mitigation.
Key Machine Learning Algorithms in Finance
Machine learning algorithms are at the core of many financial applications, helping to solve various problems and make data-driven decisions. Here are some of the main machine learning algorithms used in the financial industry, along with their applications:
Regression Analysis:
Regression analysis is widely used in finance to model and predict numerical values. For instance, linear regression can be applied to predict stock prices, where historical price data is used to establish a relationship between independent variables (e.g., time, trading volume) and the stock's price.
Neural Networks (Deep Learning):
Deep learning, a subset of neural networks, is used for various tasks in finance. Recurrent Neural Networks (RNNs) are employed for time series forecasting, while Convolutional Neural Networks (CNNs) can analyze financial documents, such as annual reports, to extract information for decision-making. Moreover, deep learning models are applied in algorithmic trading to capture complex patterns in market data.
Decision Trees:
Decision trees are used for risk assessment and investment decisions. For credit scoring, decision trees can evaluate an applicant's attributes to determine their creditworthiness. In investment, decision trees can help choose between various assets by assessing factors like risk, return, and market conditions.
Random Forest:
Random forests are used for risk management and fraud detection. In risk assessment, they can improve the accuracy of credit scoring models by aggregating multiple decision trees. In fraud detection, random forests can analyze transaction data to identify unusual patterns and anomalies indicative of fraudulent activity.
Support Vector Machines (SVM):
SVMs are used for both classification and regression tasks. In finance, SVMs can be applied to predict stock price movements, detect fraudulent transactions, and classify assets into risk categories for portfolio management.
Clustering Algorithms (e.g., K-Means):
Clustering algorithms are used for customer segmentation and portfolio diversification. By grouping customers or assets with similar characteristics, financial institutions can tailor their offerings and strategies. K-Means clustering can help identify homogeneous groups for portfolio optimization.
LSTM (Long Short-Term Memory):
LSTMs are a type of recurrent neural network that is particularly well-suited for time series forecasting. They can be used to predict stock prices, exchange rates, and other financial indicators that exhibit temporal dependencies.
Ensemble Learning (e.g., Gradient Boosting):
Ensemble methods combine the predictions of multiple machine learning models to improve accuracy. In finance, gradient boosting is applied to build robust credit risk models and optimize investment strategies by reducing bias and overfitting.
AutoML (Automated Machine Learning):
AutoML platforms automate the end-to-end process of developing machine learning models. These platforms are increasingly used in finance for various tasks, such as credit risk assessment, asset allocation, and fraud detection.
Natural Language Processing (NLP):
NLP techniques are employed to analyze financial news, reports, and social media sentiment. They help financial professionals gauge market sentiment and make informed investment decisions.
These machine learning algorithms and techniques empower financial institutions to leverage data for improved decision-making, risk management, and efficiency across various aspects of the industry, from trading and investment to lending and compliance.
The Future of Machine Learning in Finance
The finance industry continues to evolve rapidly, and machine learning is set to play an even more significant role in shaping its future. Here are some emerging trends and innovations in machine learning within the finance sector:
Quantum Computing:
Quantum computing is on the horizon, and its immense processing power has the potential to revolutionize financial modeling and optimization. It can significantly speed up complex calculations, such as options pricing, portfolio optimization, and risk assessment.
Big Data and Alternative Data Sources:
The finance sector is increasingly relying on big data analytics and alternative data sources. Machine learning models are used to extract insights from vast and diverse datasets, including social media, satellite imagery, and internet of things (IoT) data. This helps in making more informed investment decisions and improving risk management.
Explainable AI (XAI):
The need for transparent and interpretable machine learning models is growing. XAI techniques are being developed to provide clear explanations of how models arrive at their decisions, ensuring regulatory compliance and fostering trust among stakeholders.
AI-Driven Regulatory Compliance:
Machine learning is being used to streamline and automate compliance with financial regulations. AI-driven tools help financial institutions monitor transactions, detect anomalies, and ensure compliance in a more efficient and accurate manner.
Robotic Process Automation (RPA):
RPA, often combined with machine learning, is automating routine, rule-based tasks like data entry, document processing, and customer onboarding. This enhances operational efficiency, reduces costs, and minimizes errors.
Evolving Investment Strategies:
Machine learning is driving the development of increasingly sophisticated investment strategies. Robo-advisors are expected to become more personalized and capable of responding dynamically to market conditions. Reinforcement learning models are exploring new avenues in asset allocation.
Risk Management:
Machine learning models are becoming more adept at identifying emerging risks and predicting market volatility. These technologies are helping financial institutions build robust stress-testing models and adapt to unforeseen market events.
Algorithmic Trading:
Machine learning in algorithmic trading is advancing, with more advanced strategies taking into account not only historical data but also real-time sentiment analysis, news, and macroeconomic factors.
Deep Learning for Anomaly Detection:
Deep learning, especially deep autoencoders and recurrent neural networks, is being used to develop advanced anomaly detection systems. These systems can identify unusual patterns in financial data, helping detect fraudulent activities and cybersecurity threats.
In the coming years, machine learning's role in finance is likely to expand, with an increasing focus on transparency, ethical AI practices, and robust regulatory compliance. Additionally, the integration of quantum computing and the utilization of big data will bring unprecedented opportunities for innovation, enabling financial institutions to stay competitive and make more data-informed decisions. The finance industry is set to transform investment strategies and risk management, providing more tailored and resilient solutions to meet the ever-changing demands of the global economy.
Getting Started with Machine Learning in Finance
For individuals or organizations looking to incorporate machine learning into their financial operations, here's some guidance:
Understand the Basics:
Start by learning the fundamentals of machine learning. Familiarize yourself with concepts like supervised and unsupervised learning, regression, classification, and clustering. You can find online courses and tutorials on platforms like Coursera, edX, and Khan Academy.
Gain Financial Knowledge:
A strong understanding of financial principles is essential. Learn about financial markets, investment strategies, risk management, and regulatory compliance in the finance sector. This knowledge will help you apply machine learning effectively in financial applications.
Learn Machine Learning Libraries:
Python is the most popular programming language for machine learning in finance. Get proficient in machine learning libraries like scikit-learn, TensorFlow, and PyTorch. These libraries offer a wide range of pre-built algorithms and tools.
Explore Financial Datasets:
Access publicly available financial datasets to practice your machine learning skills. Platforms like Quandl, Yahoo Finance, and Alpha Vantage provide historical financial data for free. Kaggle is a great resource for financial datasets and machine learning competitions.
Online Courses and Books:
Consider enrolling in courses that focus on machine learning in finance. There are specialized programs such as the "AI in Finance" course by New York University and "Machine Learning for Trading" by Georgia Tech on Coursera. Books like "Machine Learning for Dummies" by Mueller and Massaron provide a comprehensive introduction.
Kaggle Competitions:
Participate in Kaggle competitions related to finance. These real-world challenges allow you to apply machine learning to financial problems, gain practical experience, and learn from the community.
Implement Financial Models:
Develop your own financial machine learning models. Start with simpler tasks, such as predicting stock prices or building a credit risk model, and gradually progress to more complex applications.
Stay Informed:
Keep up with the latest developments in the field of machine learning in finance. Follow relevant blogs, research papers, and news sources. Sites like arXiv and SSRN often feature academic papers on finance and machine learning.
Mitigating Risks and Challenges:
Data Quality: Ensure the quality and accuracy of your data. Garbage in, garbage out—clean and well-preprocessed data is essential.
Bias and Fairness: Be mindful of bias in your machine learning models. Use fairness-aware algorithms and practices to prevent discriminatory outcomes.
Regulatory Compliance: Stay updated on financial regulations and ensure your machine learning models comply with legal requirements.
Model Interpretability: Use models that offer explanations for their decisions, especially in critical applications like credit scoring.
Cybersecurity: Invest in robust cybersecurity measures to protect your financial data and machine learning models from malicious attacks.
Human Oversight: While automation is valuable, retain human oversight for decision-making, especially in critical financial decisions.
Incorporating machine learning into financial operations can be a transformative step, but it requires a solid foundation in both finance and machine learning. Learning from online resources, leveraging financial datasets, and participating in competitions are excellent ways to start. Additionally, be aware of the challenges and risks associated with adopting machine learning and take steps to mitigate them to ensure a successful integration into your financial operations.
Case Studies and Success Stories
Case Study 1: JPMorgan Chase
Application: Algorithmic Trading
Success Story:
JPMorgan Chase, one of the largest financial institutions globally, has successfully integrated machine learning into its trading operations. They've employed deep learning techniques to analyze market data and make high-frequency trading decisions. This initiative has led to a significant improvement in trading performance. In an interview, Pankaj Gupta, Head of Data Science for JP Morgan's Corporate and Investment Bank, noted, "Machine learning enables us to identify opportunities in the market with greater accuracy and speed. It has become an indispensable tool for our trading desks."
Case Study 2: LendingClub
Application: Credit Risk Assessment
Success Story:
LendingClub, a peer-to-peer lending platform, utilizes machine learning to assess the credit risk of loan applicants. By analyzing a multitude of borrower attributes and historical data, they've improved their lending decisions. According to Scott Sanborn, CEO of LendingClub, "Machine learning has allowed us to make more precise and faster lending decisions, leading to a reduction in default rates and an increase in investor returns."
Case Study 3: Bridgewater Associates
Application: Investment Strategy
Success Story:
Bridgewater Associates, one of the world's largest hedge funds, utilizes machine learning to enhance its investment strategies. Ray Dalio, founder of Bridgewater, emphasizes the role of machine learning in portfolio optimization. He states, "Machine learning helps us continually refine our investment strategies, adapt to changing market conditions, and achieve consistent returns for our clients."
Case Study 4: ZestFinance
Application: Credit Scoring
Success Story:
ZestFinance is a fintech company that specializes in credit risk assessment. They use machine learning to provide more accurate credit scores to underbanked populations. Douglas Merrill, CEO of ZestFinance, emphasizes the social impact, saying, "Machine learning allows us to offer fairer and more inclusive access to credit, helping those who are often overlooked by traditional scoring methods."
Case Study 5: Capital One*
Application: Customer Service Chatbots
Success Story:
Capital One has integrated machine learning into its customer service operations by using chatbots. These AI-driven chatbots handle customer inquiries, provide information on accounts, and assist with transactions. This has significantly reduced customer service response times and improved customer satisfaction. According to Tom Poole, SVP of Digital at Capital One, "Our chatbots have not only streamlined customer service but also allowed our human agents to focus on more complex issues, resulting in higher customer loyalty."
These case studies demonstrate how machine learning is being applied across the finance industry with remarkable success. The quantifiable benefits include improved trading performance, reduced default rates, enhanced investment strategies, and more inclusive credit access. These real-world examples underscore the growing significance of machine learning in financial operations and decision-making.
Conclusion
In conclusion, the integration of machine learning into the finance sector is poised to revolutionize the industry. Here are the key takeaways from this article:
Transformative Potential: Machine learning is reshaping finance by offering powerful tools for data-driven decision-making, risk management, and automation. It can enhance investment strategies, streamline operations, and provide a competitive edge in a data-rich financial landscape.
Diverse Applications: Machine learning finds applications in algorithmic trading, credit risk assessment, fraud detection, customer segmentation, portfolio management, and more. It's not limited to a single niche but has a broad spectrum of applications across the finance sector.
Real-World Success Stories: Leading financial institutions like JPMorgan Chase and LendingClub have harnessed machine learning to achieve tangible benefits, including improved trading performance, reduced default rates, and faster lending decisions.
Challenges and Risks: While the potential of machine learning in finance is vast, there are challenges such as data privacy concerns, model interpretability, and regulatory compliance. Organizations must navigate these challenges carefully.
The Future: The future of machine learning in finance holds promise, with emerging trends in quantum computing, big data, explainable AI, and enhanced investment strategies. Machine learning is set to redefine risk management, compliance, and customer service in the finance sector.
As we look ahead, it's clear that embracing machine learning in finance is not just an option but a necessity. Financial institutions, professionals, and enthusiasts should explore and embrace the possibilities offered by this transformative technology. By understanding its potential, staying informed about developments, and addressing challenges effectively, they can position themselves at the forefront of innovation in the dynamic world of finance.
Comentarios